Learn Smarter, Not Bigger: How DeepSeek is Challenging AI's Conventional Wisdom
A lot has been written about DeepSeek recently - what the company did, what it did not, why it changes everything or nothing, and also why it made many stocks drop. I want to get deeper into some non-obvious driving forces that are questioned, and explain them for a non-technical audience. Understanding these will help to make sense of why this whole discussion creates such a buzz in the AI domain.
Learning by growing
In the early 2010s, the AI models that focus on identifying objects on images were challenged to tell cats from dogs – which are very basic challenges. With more performant computer hardware, AI researchers discovered new tools to leverage that performance to improve their AI models. This is where the so-called “scaling law” was born: bigger AI model + more training data + more computer hardware to train on = better AI model.
This approach enabled AI to not only tell cats from dogs on images, but recognize a huge variety of object on images. Further, it has shaped how tech companies approach AI development for Large Language Models (LLMs). Ultimately, it enabled the highly-capable AI tools we have access to today.
Moreover, it's why we've seen a race to build ever-larger models: from GPT-3 with 175 billion parameters to GPT-4 with an estimated 1.8 trillion. The logic seemed clear - if you want breakthrough performance, you need massive investments in hardware, data centers, and computing power.
GenAI hype
With the launch of ChatGPT in late 2022, the non-tech world became aware of these capabilities, and possibilities that latest LLMs bring. In parallel, the attention of venture capital on this domain brought billions of dollars as investments into tech companies with AI focus. This money was invested in creating larger models and computational infrastructure to drive them.
How did DeepSeek contribute to the GenAI hype? Let’s look at it from another perspective. If you, as a human, want to learn a new topic, you look for the best books and videos to dive deep into the relevant aspects. You start with selecting the best learning material to acquire knowledge efficiently.
DeepSeek focused on improving how to learn. They applied some tricks to process the learning material more efficiently. Moreover, an improved learning strategy made them use less brain capacity. Thus, they made the AI learn faster and more efficiently.
However, you can also acquire new knowledge by reading through all articles on Wikipedia. You will acquire the specific knowledge you aim for in this way as well – but you will learn so much more than just this topic. This is what the big LLMs from OpenAI & other tech companies do. This is why you can use them to chat about everything.
What it means for businesses
In short, you can say that leading tech companies learn as much as they can, which requires a lot of investments. DeepSeek, in turn, focused on becoming great at specific aspects with as little investments as possible. The buzz around DeepSeek does not mean they created an AI model that is better at everything than the ones from OpenAI, Meta, Google & co. However, it highlights that there are more ways to optimize LLMs to your specific needs than just making models bigger and more expensive.
This insight got aware to a broader audience when DeepSeek released their AI model. As this undermines the foundations of the Scaling Law, the tech companies (and especially their stocks) came under pressure.
There is not the one good answer which LLM is best for a use case – it always depends on the details. But it is good to remember that there are plenty of options, many of which are cheaper than defaulting on using the latest and biggest LLMs.
The bigger picture
Does this mean that the billions of investments into generative AI are becoming useless? No, it doesn’t.
Nobody can tell whether the tech companies that grew throughout the AI hype will still exist in three years. What will stay, however, is the computational infrastructure that has been set up.
We will find many novel applications that will be powered by generative AI beyond processing text in chatbots. As an example, one glimpse into the future is given by researchers using AI to understand better how proteins work in the human body. The point is: breakthrough progress through AI will still be driven by large computational infrastructure which costs much money.
All in all: DeepSeek’s innovation highlights ways how companies can leverage existing AI models better and cheaper today. But it does not mean that future innovations don’t require large investments by companies and countries.
Want to understand more about how generative AI works and what drives their development? Check out my book "Making Sense of Generative AI" where I break down these concepts in detail in simple terms. If you're trying to separate AI hype from reality in your decisions, the book offers clear guidance through complex topics like how generative AI really works, what makes it different from traditional AI, and how to identify which use cases will create lasting value.
Find it at Amazon or Apple Books.
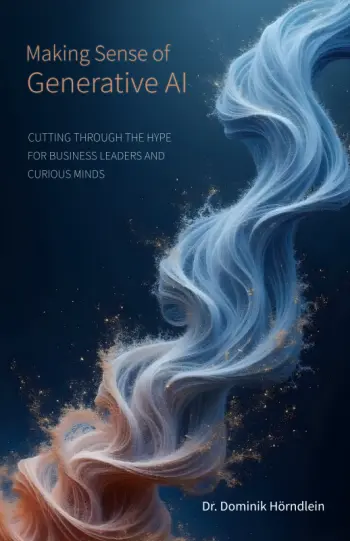